In predictive analytics what function does AI serve?
Introduction to Predictive Analytics and AI
Predictive analytics has revolutionized the way businesses make critical decisions by leveraging the power of artificial intelligence (AI). In predictive analytics what function does AI serve?
Through the analysis of vast datasets, AI uncovers intricate patterns that might elude human cognition. In the realm of predictive analytics, AI acts as the catalyst that propels businesses toward making informed and strategic choices, ultimately driving growth and success.
AI’s Role in Predictive Analytics
AI functions as a versatile tool, enabling businesses to predict various crucial aspects such as customer behavior, market trends, and potential risks.
By employing AI, businesses can forecast customer churn, pinpoint fraudulent activities, and estimate the demand for products and services, thereby optimizing resource allocation and achieving organizational objectives efficiently.
Understanding the Importance of AI in Business Decisions
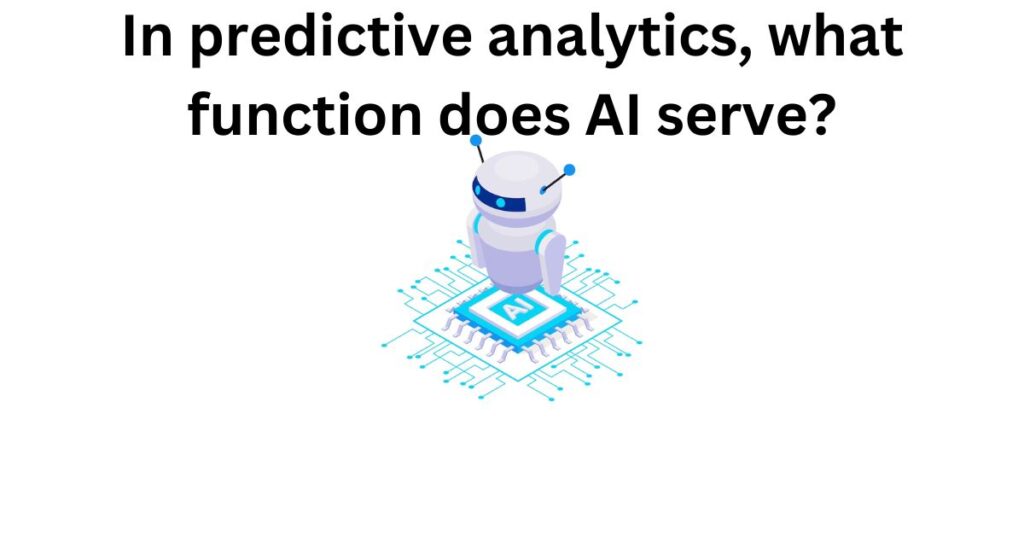
The integration of AI in predictive analytics empowers enterprises to comprehend complex data patterns, facilitating more accurate decision-making processes.
By discerning correlations and trends that are beyond human capacity, AI aids businesses in devising proactive strategies that align with dynamic market demands, ensuring sustained competitiveness and growth.
Major Applications of AI in Predictive Analytics
AI finds multifaceted applications in predictive analytics, spanning diverse sectors such as marketing, finance, and supply chain management.
It facilitates precise sales forecasting, aids in risk management, and assists in devising targeted marketing campaigns.
Moreover, AI optimizes inventory management and enhances customer relationship management, bolstering overall operational efficiency and profitability.
What is the main AI use case in cybersecurity?
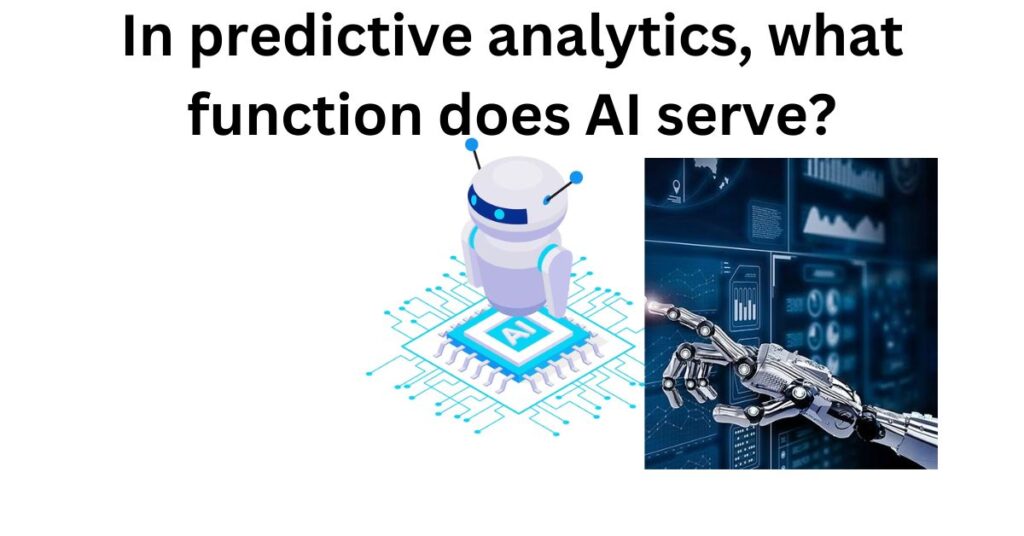
In the realm of cybersecurity, AI assumes a pivotal role in fortifying digital defenses against a myriad of evolving threats.
By meticulously analyzing extensive datasets comprising network traffic and security logs, AI identifies anomalous activities and potential vulnerabilities, enabling swift detection and prevention of cyberattacks.
The Significance of AI in Cybersecurity
The profound significance of AI in cybersecurity lies in its capacity to proactively shield systems from sophisticated cyber threats.
continuously monitoring and analyzing data, AI can swiftly recognize patterns indicative of malicious activities, empowering organizations to fortify their security protocols and thwart potential breaches effectively.
Key Applications of AI in Cybersecurity
AI serves as a robust shield against a spectrum of cyber threats, including malware, phishing attacks, and data breaches.
Its advanced algorithms can identify suspicious patterns in real-time, predict potential threats, and facilitate the formulation of preemptive security strategies.
Furthermore, AI aids in enhancing incident response mechanisms, ensuring rapid mitigation of cybersecurity risks.
Which sentence best describes reinforcement learning?
Reinforcement learning constitutes a subset of machine learning wherein an ‘agent’ learns to navigate and operate within an environment through trial and error.
By receiving rewards for favorable actions and penalties for unfavorable ones, the agent gradually adapts its behavior to maximize rewards and minimize penalties over time.
Characteristics of Reinforcement Learning
Central to reinforcement learning is the process of continuous learning and adaptation. Through trial and error, the agent comprehends the consequences of its actions, learning to prioritize actions that lead to positive outcomes while avoiding those resulting in negative consequences.
This iterative learning process fosters the development of robust decision-making capabilities within the AI system.
Real-World Applications of Reinforcement Learning
The practical applications of reinforcement learning extend across diverse domains, ranging from robotics and autonomous vehicles to industrial automation and game development.
For instance, in the context of robotics, an AI-powered robot can learn complex tasks such as locomotion or manipulation through continuous trial and error, gradually refining its movements to achieve predefined objectives.
Understanding the Potential of Reinforcement Learning
Reinforcement learning exhibits immense potential in training AI systems to undertake intricate tasks that demand adaptive decision-making.
Its ability to learn from experiences and optimize actions based on predefined objectives positions it as a crucial tool for developing intelligent systems capable of operating in dynamic and complex environments.
Benefits and Limitations of Reinforcement Learning
While reinforcement learning empowers AI systems with adaptive decision-making capabilities, its implementation entails certain challenges.
Despite its potential, the slow learning process and high computational costs associated with reinforcement learning necessitate efficient algorithm design and resource management to ensure optimal performance in real-world scenarios.
Examples of Reinforcement Learning in Action
Consider a scenario where a robotic system is learning to perform a specific task, such as navigating through a complex terrain.
Through the process of trial and error, the AI-powered robot receives rewards for successful movements towards the target and penalties for deviating from the desired path.
Over time, the robot learns to optimize its movements, effectively navigating the terrain and reaching the predefined destination.
Challenges in Implementing Reinforcement Learning
The successful implementation of reinforcement learning hinges on addressing various challenges, including the need for substantial computational resources, meticulous algorithm design, and the management of complex decision-making processes.
Ensuring the seamless integration of reinforcement learning in diverse real-world applications demands a comprehensive understanding of its limitations and the development of tailored solutions to mitigate potential setbacks.
Conclusion
As AI continues to evolve, its indispensable role in predictive analytics and cybersecurity remains pivotal. By harnessing the power of AI-driven predictive insights and reinforcing digital defense mechanisms, businesses can proactively adapt to dynamic market landscapes and fortify their cybersecurity posture.
Moreover, the transformative potential of reinforcement learning underscores the significance of continuous innovation in the development of intelligent systems capable of navigating complex real-world challenges.
FAQs
- How does AI enhance predictive analytics efficiency? AI enhances predictive analytics efficiency by processing vast datasets and identifying complex patterns that drive informed decision-making.
- What are the key benefits of AI integration in cybersecurity? The key benefits of AI integration in cybersecurity include swift threat detection, proactive risk management, and the formulation of preemptive security strategies.
- Can reinforcement learning be applied to industrial automation? Yes, reinforcement learning can be applied to industrial automation to facilitate adaptive decision-making in complex manufacturing processes.
- What are the primary challenges associated with implementing reinforcement learning? The primary challenges associated with implementing reinforcement learning include high computational costs, slow learning processes, and the complexity of decision-making in dynamic environments.
- How does AI contribute to the advancement of autonomous vehicles? AI contributes to the advancement of autonomous vehicles by enabling adaptive decision-making and real-time analysis of complex driving environments, ensuring safe and efficient navigation.